Background
Avalanche risk assessments in alpine regions critically depend on detailed information about snowpack conditions. Traditional methods, including weather forecasts, manual observations, and snow pit analyses, provide valuable insight but are limited in their ability to capture the high spatial variability often observed within the snowpack.
This oversight can compromise avalanche forecasting accuracy, while climate change further increases the demand for accurate snowpack monitoring and avalanche mitigation. To address the current limitations, GEORESEARCH, a non-profit research institution based in Austria, conducted surveys using UAV-based ground-penetrating radar (GPR) technology.
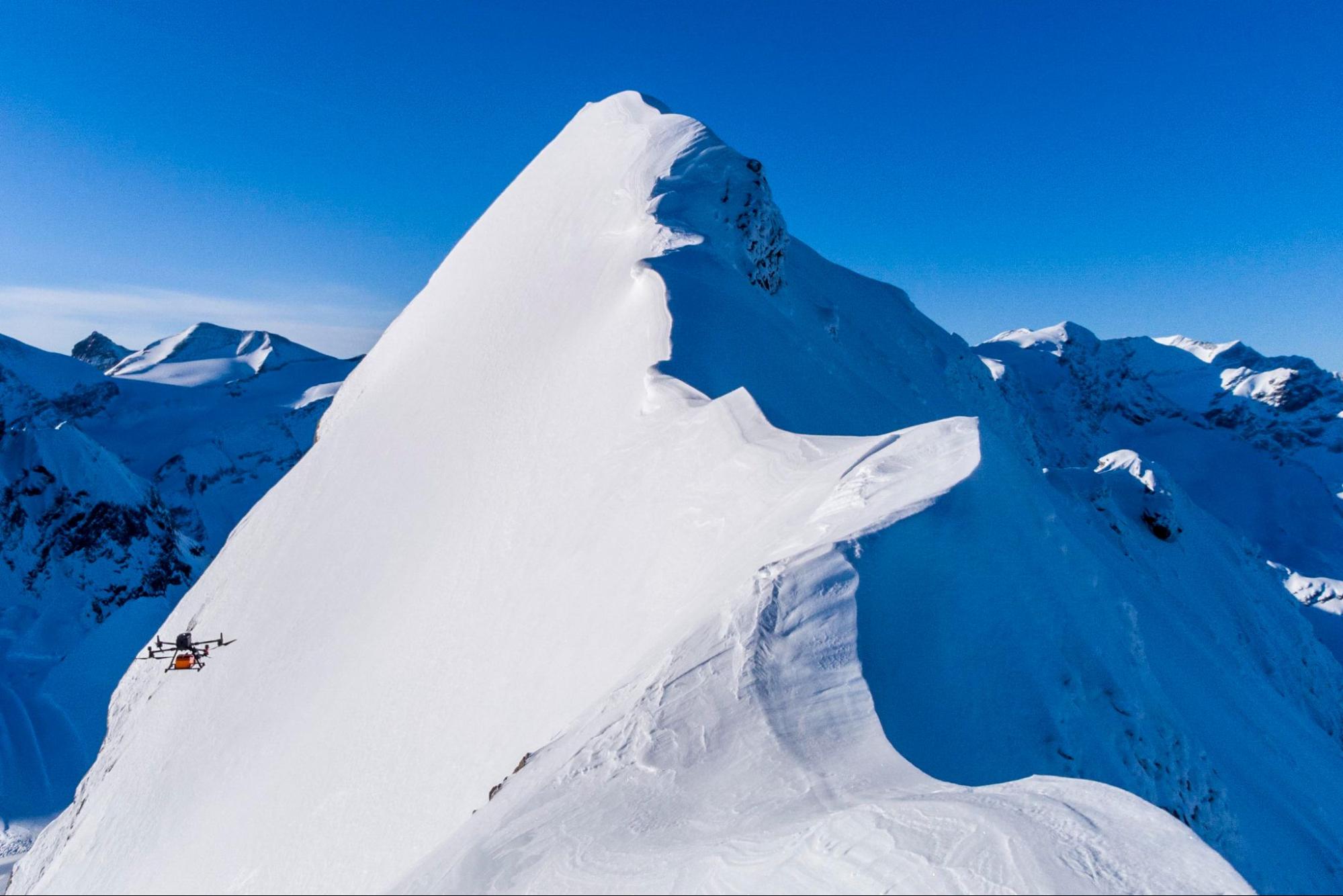
Challenges
Current methods for assessing snowpack conditions present several difficulties:
Limited spatial resolution. Conventional methods often provide data from isolated points, missing critical variability over short distances, and failing to represent spatial patterns.
Measurement accuracy constraints. Traditional approaches such as manual snow pits and probing are labor-intensive, error-prone, and limited by human subjectivity.
Climate change impact. Rapidly changing snowfall patterns require more frequent and precise measurements to ensure accurate predictions of snowpack stability, vital for sustainable snowpack monitoring.
Solution and Key Findings
To generate detailed subsurface images of alpine snowpack, the GEORESEARCH team employed SPH Engineering’s UAV-borne ground-penetrating radar (GPR) technology.
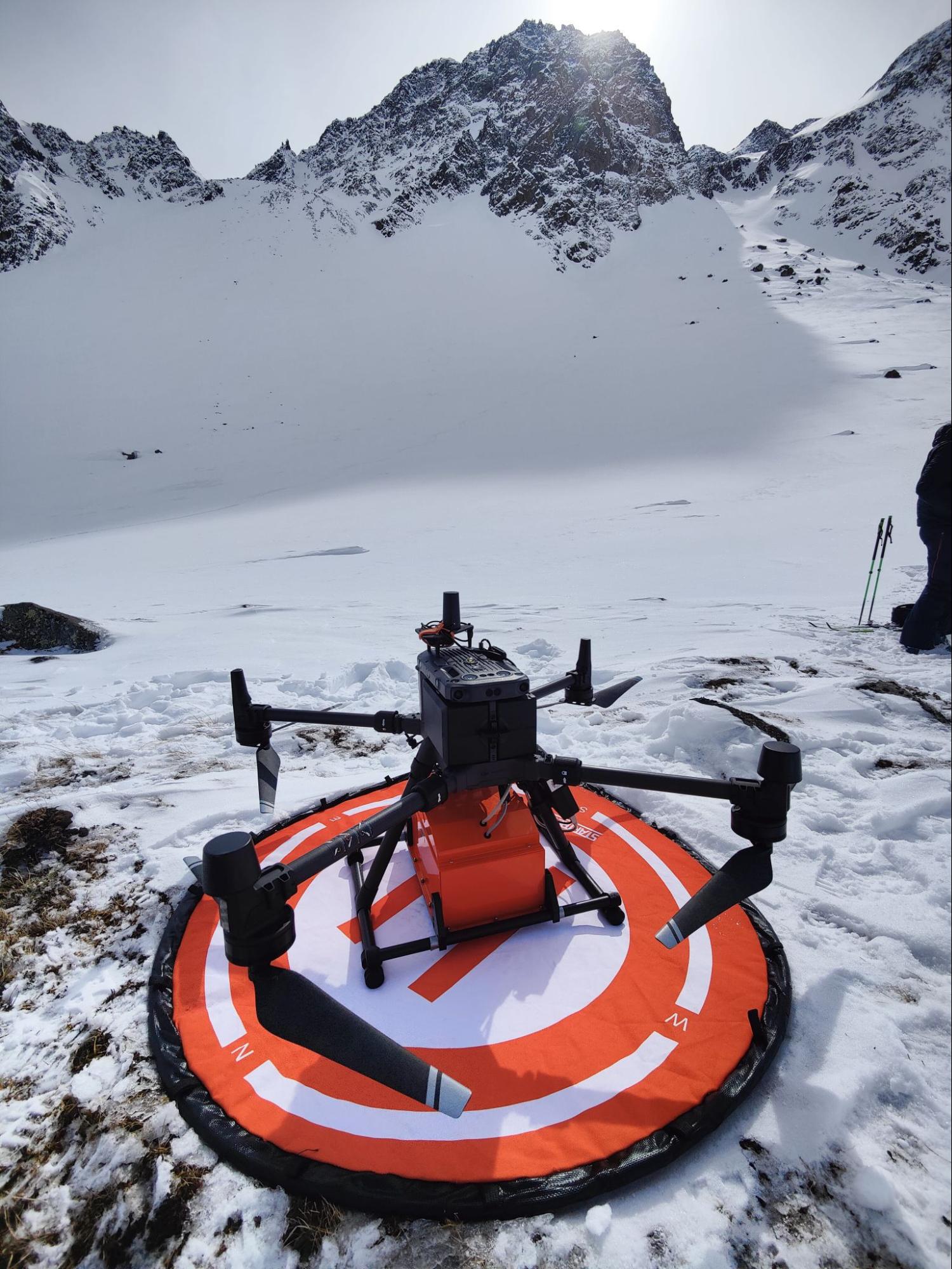
Zond Aero 1000 GPR mounted on a drone provided rapid data acquisition and broad coverage over the alpine terrain, reducing survey time and increasing coverage area.
At Stubai Glacier, the GPR revealed substantial variability in snowpack depth, identifying significant fluctuations within a small area of just 0.07 km². Depth measurements ranged dramatically — from less than 1 m to over 4 m. This detailed spatial data has the potential to improve local avalanche risk assessments, allowing for more accurate predictions of potential hazards.
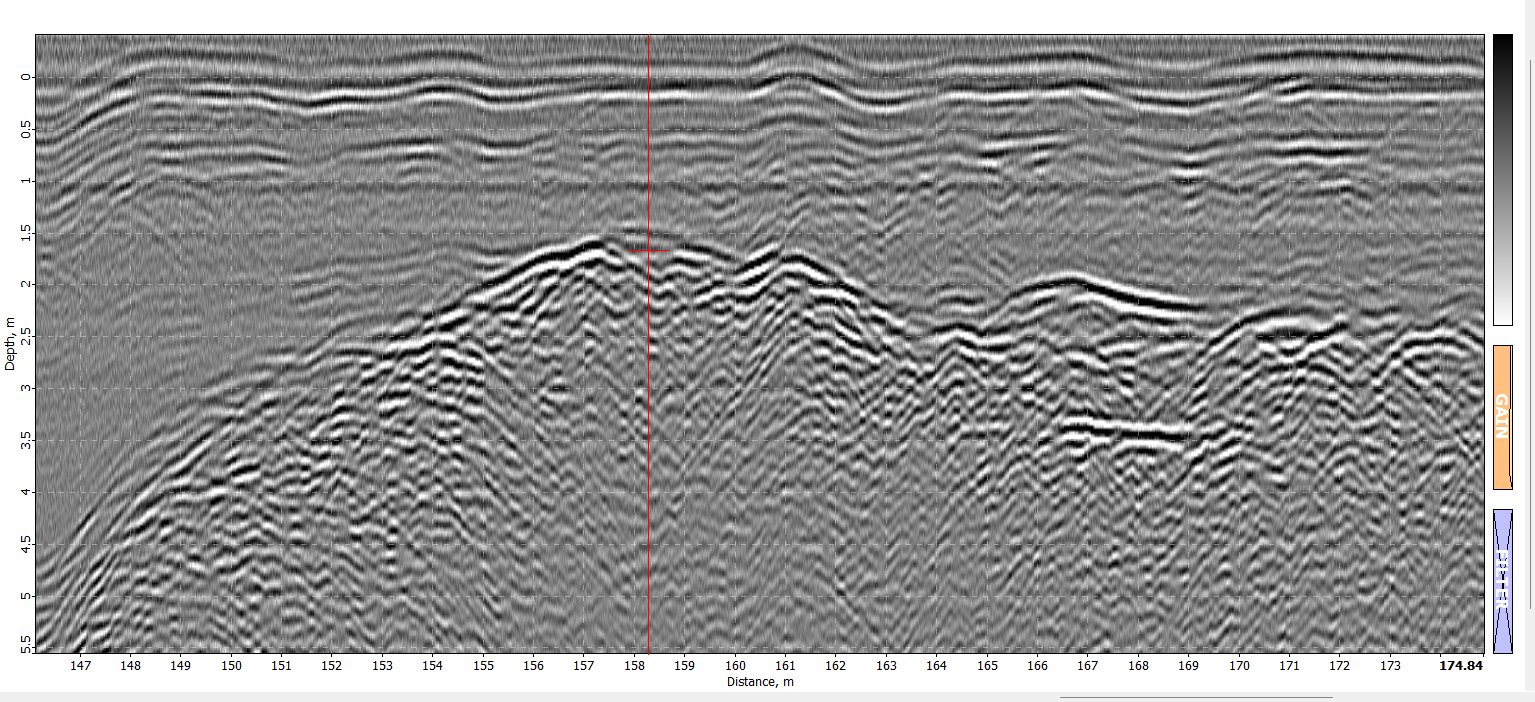
Notably, a high correlation (R²=0.93, r=0.97) was found between UAV-borne GPR measurements and conventional probe measurements, showcasing the method's reliability for snow depth assessment.
Conclusion
The results of this study demonstrate that UAV-borne GPR technology effectively maps snowpack heterogeneity at a high spatial resolution. The strong correlation with traditional snow depth measurements proves the reliability of this approach.
By unveiling hidden spatial variabilities, SPH Engineering’s integrated system enables more informed decision-making in avalanche forecasting and snow distribution patterns across alpine terrains. Furthermore, the use of readily available UAV platforms ensures that this methodology is not only effective but also scalable and accessible for future snowpack studies.