Drone technologies from SPH Engineering allowed to improve data collection and processing to 50 ha/day from manually collected 2.5 ha/day.
The knowledge of how many plants have been planted and how they are growing is key to optimizing labor and efficient use of resources in agriculture. The exponential growth of new technologies, specifically Remotely Piloted Aircraft Systems (RPAS), also known as drones equipped with sensors for data collection, have become work tools that allow fast and profitable data collection in agricultural production property. Despite the enormous benefits that the implementation of technology in agricultural production brings, it is necessary to guarantee that the data obtained through the use of images captured by the sensors installed in the drones is transformed into operational space products, resulting in data that provides an idea of the spatial distribution and the number of plants planted on the properties.
Therefore, in order to help producers improve production processes through the implementation of these technologies; a research project by Andrés Castillo from Agrointeligencia Geoespacial (Costa Rica) was conducted, which bears the name of “State-of-the-art Convolutional Neural Network applied in the automatic detection of pineapple plants from RPAS images”. The data used in the research was obtained from one of the properties of the producer members of La Cámara de Piñeros Unidos de Costa Rica (Committee of United Pineapple Sowers of Costa Rica), in an area planted with young plants of at least two weeks, located in the canton of Río Cuarto of Alajuela province.
‘The manual process of counting plants was carried out by qualified and trustworthy personnel, who, through the use of a mechanical counter, also known as "Maria", count each plant found in the fields. Weekly, the producers sow an average of 15 hectares per day, each hectare has seventy-two thousand plants, which must be counted by the staff in a week. On average, a man's yield per day is 2 to 2.5 hectares counted per day (2.5 ha/day)’, Andres Castillo Leiton, Agrointeligencia Geoespacial, explains.
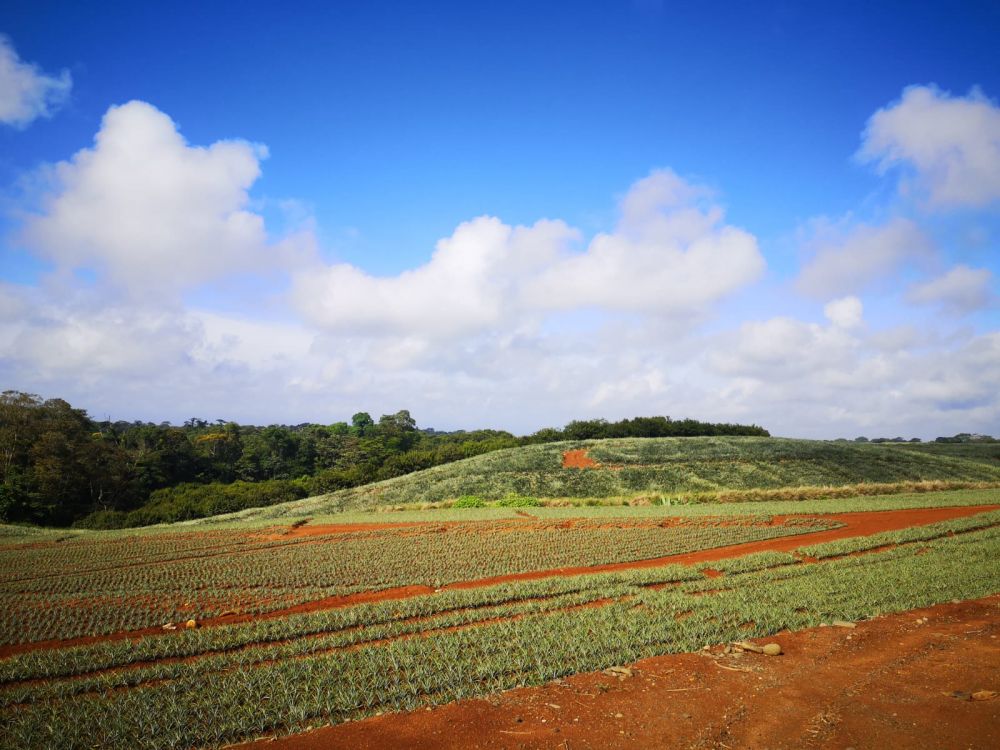
‘For the adequate generation of the orthophoto, it is necessary to use a drone with a 1” sensor of at least 20-megapixel resolution, flying at a height between 45 meters and 60 meters in order to obtain a ground sampling distance or GSD between 1.6-2 cm/pixel. For the current study, a DJI Phantom 4 Pro drone was used and the flight was planned using the UgCS software, where the horizontal and vertical overlap was configured to be 85% at a flight height of 45 meters. With this configuration, a yield of 50 hectares per day (50ha/day) was obtained with a drone. In comparison, on average, a man's yield per day is 2 to 2.5 hectares counted per day (2.5 ha/day)’, Andres adds.
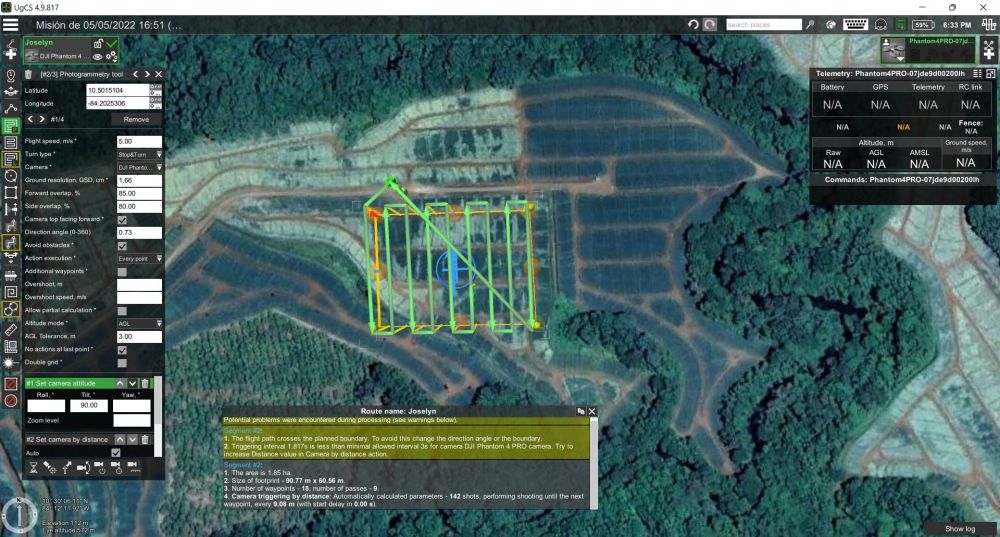
As a part of SPH Engineering Consulting and Development activities, the company’s engineers supported the implementation of the processing workflow. The processing pipeline consisted of the following steps:
- Gathering and preparing a training image set
- Training an object detection model for pineapples
- Building an algorithm for automatic result separation by field boundaries
In general, the process of obtaining the images up to the final report of the detected plants can be carried out in 24 hours, which represents a processing yield of 50* ha/day, significantly reducing costs for the producer when it is compared to the manual process.
According to Andres, fast and proper plant counting brings the following benefits to the grower:
- Verify the number of planted plants indicated by the contracted company versus the plants in the field, in order to make the correct payment.
- Verify if the dosage of agrochemicals is adequate to avoid an overdose.
- Generate projections of what has been sown and the harvest that is intended to obtain.

'From the analysis of the research results, it was concluded that artificial intelligence and images captured with drones can be used to accurately monitor crops and count plants, specifically pineapple plants, and that allows the producer to store acquired information in a geospatial database for decision making. Despite this, it is important to highlight that in order to obtain the expected results, conditions such as a ground sampling distance must be met, which allows the artificial intelligence algorithm to detect the plants in the images and that there are no dodder weeds within the plantations since these can generate misinformation', Andres concludes.